Introduction
Artificial Intelligence (AI) is rapidly transforming nearly every scientific domain, and genomics—the study of genes and genomes—is no exception. AI-driven genomics refers to the integration of advanced machine learning and AI algorithms with genomic science to decode, analyze, and interpret the vast and complex data embedded in DNA. This fusion is revolutionizing healthcare, personalized medicine, agriculture, and evolutionary biology.
By automating data analysis and discovering patterns too complex for human recognition, AI is enabling faster, more accurate insights into gene function, mutations, and disease mechanisms. In a world inundated with genetic data, AI is not just a tool—it’s an essential partner in the future of biological discovery.
Understanding Genomics
Genomics is the branch of biology that focuses on the structure, function, evolution, mapping, and editing of genomes—all of the DNA within a single cell of an organism.
Key Aspects of Genomics:
- DNA Sequencing: Determining the precise order of nucleotides in DNA.
- Gene Annotation: Identifying gene locations and functions within the genome.
- Comparative Genomics: Studying genetic similarities and differences between species.
- Functional Genomics: Linking gene functions to phenotypes and biological processes.
With the advent of Next-Generation Sequencing (NGS), massive amounts of genomic data are generated. But analyzing this deluge of information manually is nearly impossible—this is where AI steps in.
What Is AI-Driven Genomics?
AI-driven genomics applies artificial intelligence technologies—especially machine learning (ML), deep learning (DL), and natural language processing (NLP)—to process, interpret, and make predictions based on genomic data.
AI helps in:
- Identifying disease-associated genes
- Predicting the effects of mutations
- Understanding gene expression patterns
- Accelerating drug discovery and development
- Enhancing personalized medicine approaches
This synergy accelerates discoveries that would otherwise take decades and enables precision healthcare tailored to individual genetic makeups.
Core Technologies Used
1. Machine Learning (ML)
AI algorithms learn from historical genetic data to detect patterns or predict gene-disease relationships.
2. Deep Learning
Used for analyzing high-dimensional data such as DNA sequences or gene expression profiles through neural networks.
3. Natural Language Processing (NLP)
Helps mine scientific literature, extract relevant genetic information, and annotate genes using language-based data.
4. Bioinformatics and Computational Biology
AI enhances traditional bioinformatics pipelines, improving accuracy and reducing time for genomic annotation and interpretation.
5. Cloud Computing and Big Data Platforms
Essential for storing and processing terabytes of sequencing data quickly and efficiently.
Applications of AI in Genomics
1. Disease Diagnosis and Risk Prediction
AI can:
- Detect genetic mutations linked to diseases such as cancer, Alzheimer’s, and rare genetic disorders.
- Predict disease susceptibility based on polygenic risk scores (PRS).
- Interpret variants of unknown significance (VUS) in clinical settings.
Example: Google’s DeepVariant tool uses deep learning to convert raw DNA sequencing data into highly accurate variant calls.
2. Personalized Medicine
AI helps tailor treatments based on an individual’s unique genetic profile, known as precision medicine.
- Recommends drug types and dosages
- Predicts adverse reactions
- Selects appropriate therapeutic interventions
Example: IBM Watson Genomics analyzes a patient’s DNA and suggests potential cancer therapies.
3. Drug Discovery and Development
AI speeds up drug discovery by:
- Identifying drug targets via genetic markers
- Modeling protein interactions
- Screening compound libraries for genetic relevance
Example: BenevolentAI uses AI to identify gene-drug relationships and suggest repurposed drugs.
4. Agriculture and Plant Genomics
AI aids in:
- Identifying drought- or pest-resistance genes
- Enhancing crop yield and nutritional value
- Predicting genotype-phenotype correlations in plants
Example: AI algorithms help genome-edit crops using CRISPR by targeting ideal genetic sites.
5. Population Genomics and Evolutionary Studies
AI facilitates:
- Analyzing genetic variations across populations
- Studying human migration and ancestry
- Understanding evolutionary gene functions
Large datasets like the 1000 Genomes Project and GenomeAsia 100K are powered by AI analytics to uncover ethnic and population-specific genetic traits.
AI-Driven Genomics in India
India has vast genomic diversity and a growing AI ecosystem. Institutions and initiatives are merging these domains to revolutionize healthcare and agriculture.
Major Initiatives:
- Genome India Project: Sequencing 10,000 Indian genomes for health and disease research.
- INDiGen Programme (CSIR): Decoding Indian genomes to aid in precision medicine.
- AI for Genomics Challenge (NITI Aayog): Encourages AI solutions for early disease prediction and treatment based on Indian genomics.
Startups like Mapmygenome, MedGenome, and Qure.ai are leveraging AI for genetic diagnostics and personalized wellness in India.
Benefits of AI in Genomics
Benefit | Description |
---|---|
Speed | AI accelerates data analysis from weeks to hours |
Accuracy | Increases the reliability of variant calling and disease prediction |
Scalability | Easily handles massive genomic datasets across global populations |
Cost Efficiency | Reduces labor and computational costs in research and diagnostics |
Real-time Monitoring | Tracks genetic changes in pathogens (e.g., SARS-CoV-2) rapidly |
Challenges and Limitations
1. Data Privacy and Ethics
- Genomic data is highly sensitive.
- Risks of misuse or breaches can violate personal and ancestral privacy.
- Requires robust genomic data protection laws, especially in developing countries.
2. Interpretability
- Many AI models (especially deep learning) are black boxes.
- Understanding why an AI made a specific genetic prediction remains difficult.
3. Bias in Data
- AI models trained mostly on Western populations may underperform on diverse ethnic groups.
- Inclusive genomic datasets are needed to ensure equity.
4. Integration into Clinical Practice
- Doctors may lack training in genomics and AI, limiting adoption.
- Regulatory frameworks for AI in clinical genomics are still evolving.
5. Infrastructure Constraints
- High computational needs and storage demands can be a barrier in resource-limited settings.
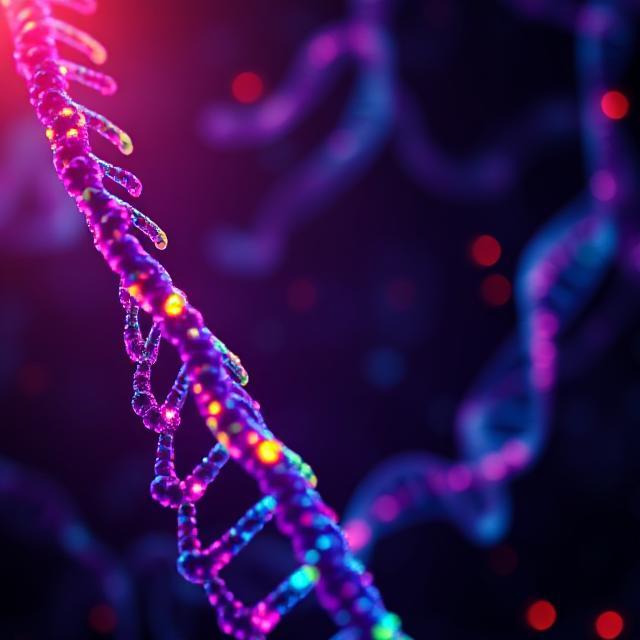
Ethical and Regulatory Considerations
- Informed Consent: Participants must understand how their genetic data will be used, especially in AI applications.
- Data Ownership: Should individuals or institutions control the genomic information?
- Algorithm Transparency: AI models should be explainable and auditable to prevent discrimination or errors.
- Policy Gaps: Developing nations often lack specific regulations for AI-genomics intersections.
Global initiatives like GA4GH (Global Alliance for Genomics and Health) advocate ethical standards and interoperability in genomic research.
Future of AI-Driven Genomics
The future looks highly promising with the following developments:
1. Real-time Genomic Monitoring
Wearable biosensors may provide continuous genomic data streams for early disease detection.
2. Automated Genome Editing
AI could design CRISPR edits autonomously, correcting mutations with pinpoint precision.
3. Digital Twins in Healthcare
Personalized genetic models of individuals will help simulate treatments before application.
4. Federated Learning
A technique where AI models learn from decentralized datasets, preserving privacy while improving predictions.
5. Multi-Omics Integration
Combining genomics with transcriptomics, proteomics, and metabolomics using AI to get a full picture of biology.
Conclusion
AI-driven genomics marks the next frontier in biological science—where data, algorithms, and human biology converge. By decoding the language of life faster and more precisely, AI is opening doors to revolutionary applications in medicine, agriculture, and evolutionary biology.
While challenges in ethics, bias, and infrastructure persist, the path forward lies in interdisciplinary collaboration, inclusive data practices, and responsible innovation. AI, when harnessed wisely, will not just read our genomes—it will help write the future of life sciences.